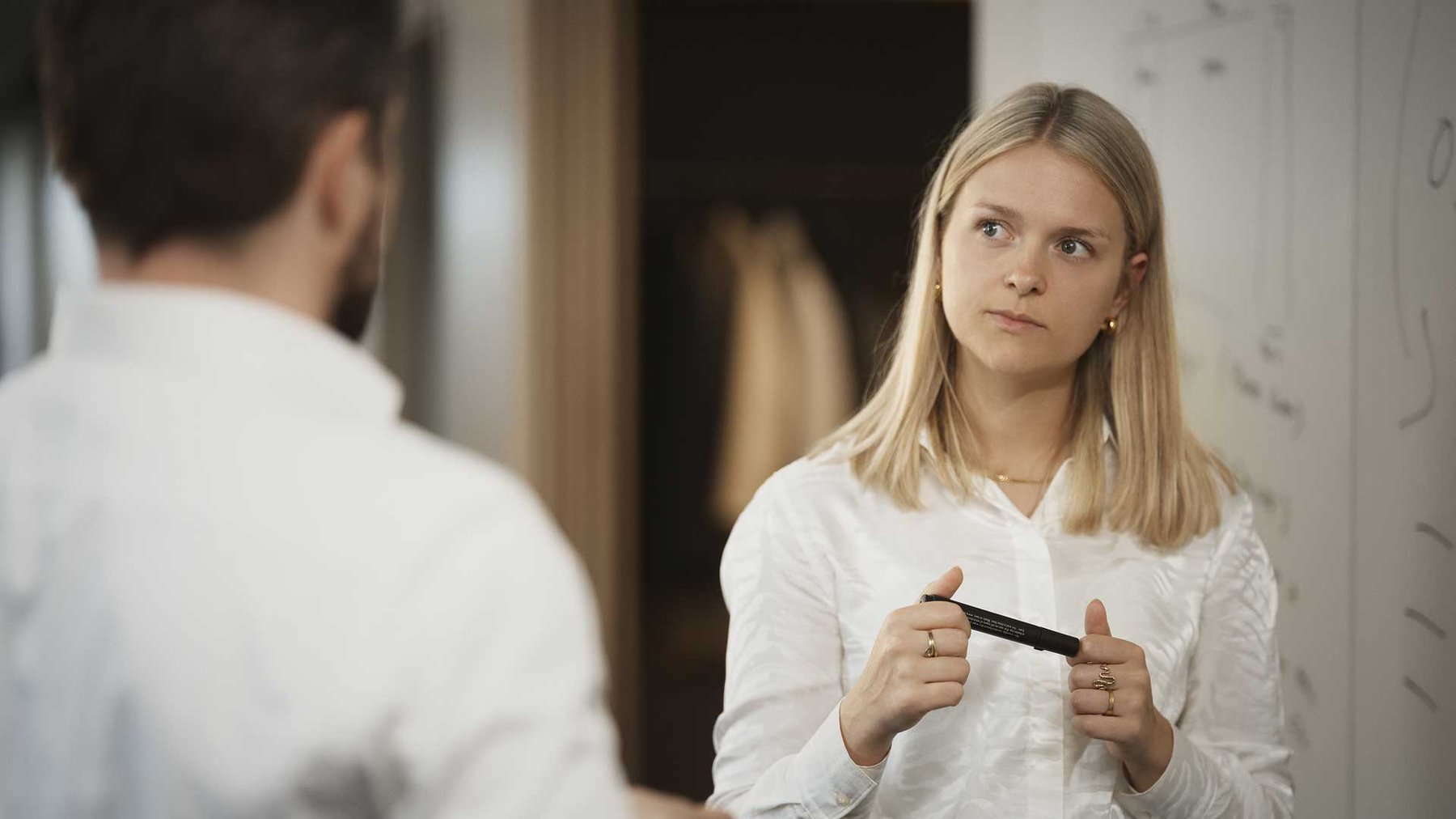
Data Warehouse
A cloud-based Data Warehouse is the starting point for good Business Analytics
Overview, structure, and validity
A central Data Warehouse is the cornerstone of our solutions. By building a robust Data Warehouse, we ensure consistent data and the ability to make meaningful comparisons.
We love building Data Warehouses
There are many requirements today with regard to a robust Data Warehouse. The number of data sources increases for most businesses, and the requirements for functionality covers a range of aspects: the history of master data and transactions, data and user-driven security, Master Data mapping and enrichment, handling of processes to improve data quality, and increased data update frequency that is in some cases nearly in real time.
We master the entire spectrum, from design of the architecture, sizing of the infrastructure, cloud, hybrid, or on-premises, integrations, and data modeling. We work with Microsoft’s ETL tools, Integration Services, and Microsoft’s Master Data tool MDS, both of which are an integral part of Microsoft’s SQL Server.
We can also help you decide whether your solution should run as an internal solution, a cloud solution, or a hybrid of both of them.
Our Data Warehouse architecture follows our twoday kapacity Best Practice method that is a method developed and adapted for new versions of SQL over the past 10 years, and it ensures consistency and rapid Time to Market for the customers’ solutions.
Master Data Management is a natural part of a Business Analytics project at twoday kapacity.
Master data management in a Business Intelligence context concerns the data dimensions where it may be necessary to develop groupings or hierarchies other than those available in the source systems. It may also be where the source systems have different structures for the same dimension data, so there is a need to map for a common reporting structure. The Data Warehouse design also handles the need to save the history of the individual master data values and the history of the hierarchies that are laid over the master data.
A Master Data Management solution in the context of Business Intelligence should also be able to list missing and erroneous attributes of newly created master data that is retrieved from the source systems, so that a master data manager, for example, receives a notification of missing values when creating customers or products. These can be created correctly thereafter in the source system and be included in the Data Warehouse after the next ETL run.
Finally, a Master Data Management solution can be used to manage target KPIs in a Performance Management set-up, allowing users to periodically adjust these targets without changing the logic in the Data Warehouse or reports.
twoday kapacity recommends Microsoft SQL Server Master Data Services, MDS, which is a part of Microsoft SQL Enterprise.
SQL Server Master Data Services (MDS) contains the following functionality:
Fully integrated best practice import/export procedure as part of the ETL process.
Advanced user security integration for updates.
Design guidelines for advanced modeling.
Functionality for automatic deployment.
MDS is the SQL Server solution for handling Master Data Management to enrich source system data or the Data Warehouse with master data.
Add-in for Excel or web application for the end-user’s update of master data attributes.
The Data Warehouse of the future is here now
The Business Analytics requirements of the future will require that we expand the traditional Data Warehouse architecture to accommodate both the reporting and the analyses that we know today as well as the data and analytical needs of the future.
- External data must be included with the company’s internal data sources.
- New data sources may have no framework so that the data can be structured, semi-structured, or unstructured.
- Companies collect new types of data from their products, shops, or from the equipment that they maintain. The data volumes can be so large that we are talking about “big data”, or they may need to be delivered and analyzed in real time to be useful.
- Businesses need insights from Advanced Analytics and, in some instances, also in real time.
- Exploratory technologies like Machine Learning are used to find new insights.
- Generally, data volumes are growing and it is essential to design the architecture accordingly.
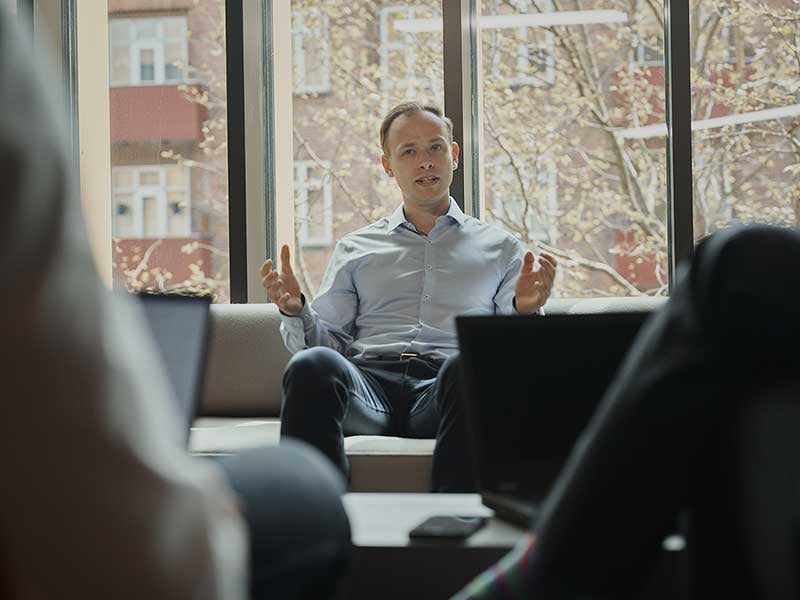
Bimodal Data Warehouse
In Gartner’s terminology, we are talking about bimodal as a prerequisite for implementing a digital transformation. Our Data Warehouse must be able to accommodate both the stable and well-described (Mode 1) and the flexible and experimental, which are targeted at the information we do not know (Mode 2). The architecture must also allow a process where the exploratory mode 2 findings can be used in classical mode 1 Data Warehouse.
Automation (BIML)
We are developing with the expectation of changes
When companies today start on their first Business Analytics project or prepare for their generation 2.0 solution, there is one factor that everyone has to work with:
Your source systems will change, they need to be upgraded or replaced, and more data sources will probably become available along the way. This means that we must build the Data Warehouse based on a principle where metadata can define our integrations, naming and, in some cases, also our logic, so that we can replace a source system and, with only a few fixes, achieve the same data contexts in our analyses as were available before the replacement.
At Kapacity, we use a best practice method that takes this into account when we build your Data Warehouse solution.
As part of our method, we use BIML (Business Intelligence Markup Language), which is a definition language that can be used to define and automate the jobs that a developer usually performs manually.
There are many advantages to BIML:
Much faster development of integrations and logic.
Faster addition of new data, both tables and columns.
Ensuring fixed standards across multiple developers and project phases.
Fewer manual errors and much easier maintenance, including in connection with replacement of source systems.
BIML generates the exact same code as manual development, so if at a later date you decide to move away from twoday kapacity’s best practice method or BIML as the definition language, the code can be modified directly.
Checklist: Activities you need to think about before, during and after your Cloud Data Warehouse project
The main driver for moving a fully or partially established Data Warehouse to a Cloud Data Platform is often the desire for a more agile approach to future changes as well as expanding the solution on both the data and user side.
We have made a checklist that deals with the activities that should be prepared before the actual development of a Cloud Data Warehouse begins. It deals with decisions about processes, roles and responsibilities, which we know from experience have a great effect on the success of the project.
You can see the headlines of the list below, and if you want to read the entire list, you can read the blogpost here.
- Establishment of a model for collecting business requirements and involving the business during the project.
- Establishment of the target architecture
- Establishment of project governance
- Establishment of design method and safety concept
- Establishment of dashboard design template based on best practice visualization theory
- Collect all activities in a roadmap
- Establishment of detailed design
- Establishment of DevOps setup
- Development
- Test
- Production setting and go-live support
- Documentation
- Project Management
- Knowledge sharing and cloud competence building
- Establishment of monitoring of the solution
- Training of users
- Establishment of support processes including roles
- Planning anchoring activities and training for new employees
- Establishment of governance for continuous development of the solution
- Establishment of roles and competencies for handling production setup
Case: Krifa reaches new heights with a modern Data Warehouse
Get inspiration for your Data Warehouse: With a modern Data Warehouse based on the latest technology, Krifa can now make better decisions based on a common ground.
The new solution creates a common data basis where the truth about data is gathered in one place. This means that different terms and concepts are agreed upon and used the same way across the organization.
The common data basis will help create insight and curiosity for the benefit of employees, members, and the community. This eases the pressure on Krifa’s BI team at the same time. In the long run, value creation will be created directly by Krifa’s employees.
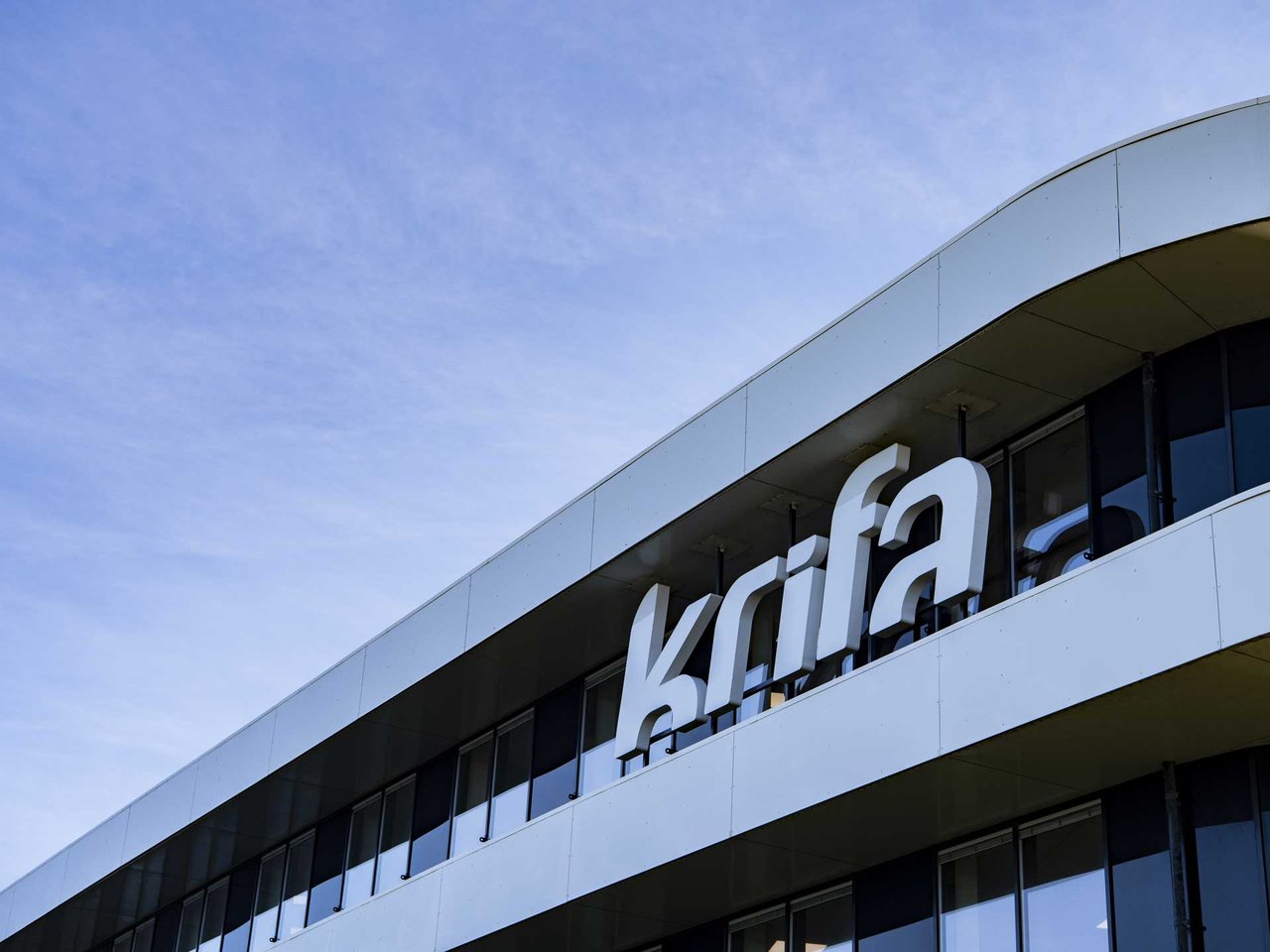
Do you want to get started? So do we
Do as a large number of the country’s most ambitious companies:
Fill out the form or get in touch with Søren – then we can have a chat about your challenges and dreams.